Aug 30, 2024
Sachin Kose Paul
In the well-known fable “The Blind Men and the Elephant,” a group of blind men encounters an elephant for the first time. Each man touches a different part of the animal and forms a distinct impression of what the elephant is like based on the part they touched.
One man touches the trunk and believes the elephant is like a thick snake.
Another touches the leg and thinks the elephant is like a tree trunk.
A third feels the ear and imagines the elephant is like a fan.
The one who touches the side thinks the elephant is like a wall.
Another touches the tail and believes the elephant is like a rope.
Their inability to see the whole elephant leads to fragmented and incomplete understandings, each shaped by their limited experience.
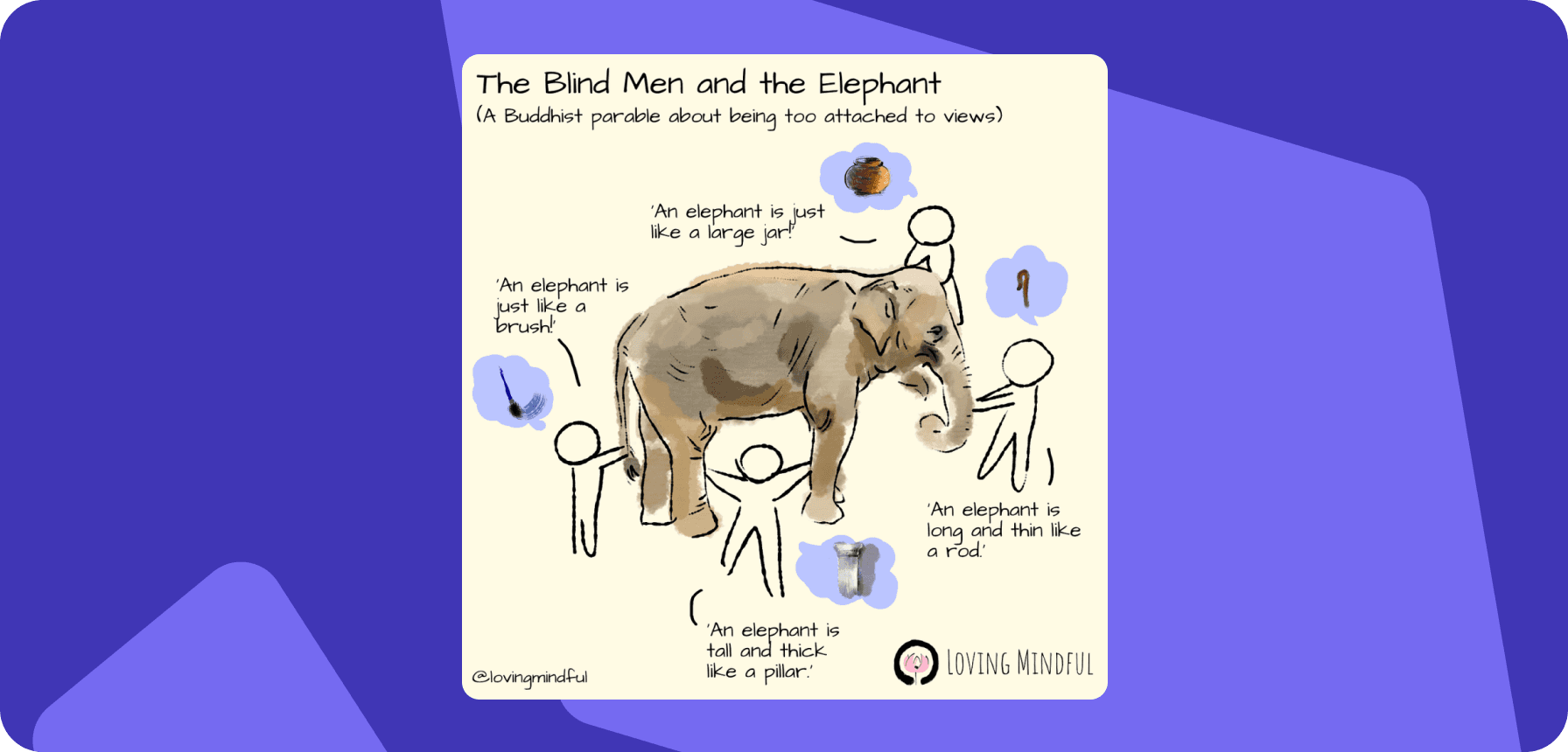
In the AI landscape, this is what we call “bias.”
AI systems are shaped by the data they are trained on, much like human perspectives are shaped by personal experiences and conditioning. Just as humans interpret the world through the lens of their individual histories and environments, AI systems interpret and make decisions based on the data and algorithms they have been exposed to.
Age, gender, and ethnicity are vulnerable categories that, if left unchecked, can result in major unintended disparities across multiple use cases. In finance, for example, biased AI could lead to unfair lending practices or discriminatory financial assessments, disproportionately affecting certain demographic groups. The consequences of such biases are profound, as evidenced by several studies that have shown how these disparities manifest in critical areas like lending, hiring, and healthcare.
The trouble doesn’t necessarily end there. In current algorithmic setups, bias is not just inherent but can also be introduced by a malicious third party by leveraging the vulnerabilities in the data as well as the model design. This underscores the urgent need to safeguard AI systems against both inherent and induced biases. As Cassie Kozyrkov humorously puts it, “We’re not wearing our seatbelts, folks.”
When developing AI systems, it is crucial to address both fairness and security concerns.
Selecting the right tools for these tasks is essential to building models that are both ethical and robust.
To identify and mitigate biases in AI, several fairness toolkits are available, each designed to ensure that machine learning models produce equitable outcomes across different demographic groups. One such toolkit, AI Fairness 360 (AIF360), developed by IBM Research, offers a comprehensive set of fairness metrics and algorithms to assess and mitigate bias. These metrics include:
Disparate Impact: Measures the ratio of favourable outcomes between different demographic groups, highlighting potential discrimination.
Equal Opportunity Difference: Evaluates whether the true positive rates are equal across groups, ensuring fairness in positive outcomes.
Statistical Parity Difference: Assesses the difference in the rate of favourable outcomes across groups, indicating potential bias.
Other notable fairness tools include Fairlearn, which provides techniques to mitigate bias during model training, and the What-If Tool, which allows for visual exploration of model outcomes and potential biases.
Alongside fairness, it’s important to consider the security of AI models, particularly their susceptibility to adversarial attacks.
Toolkits like SecML, CleverHans, and the Adversarial Robustness Toolbox (ART) are designed to simulate and defend against these attacks. SecML, for instance, focuses on adversarial machine learning, providing tools to test model robustness and develop defences against potential threats.
By integrating these toolkits into the development process, AI practitioners can create models that not only mitigate bias but also withstand adversarial manipulation, ensuring both ethical integrity and security.
“With great power comes great responsibility.” As we push the boundaries of what AI can achieve, it is crucial that we do so with a commitment to fairness, security, and transparency. By innovating with integrity, we ensure that the advancements we make not only drive progress but also uphold the values that make technology a force for good.
✅ Follow Koodoo on LinkedIn today for GenAI insights and real-world applications in financial services.